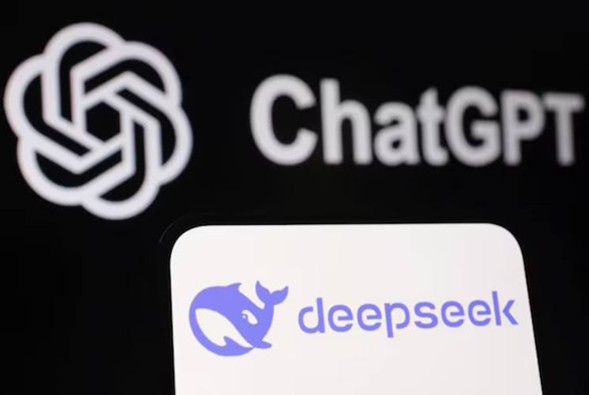
On the 21st of January, President Donald Trump announced the Stargate Project (a partnership between OpenAI, Oracle, Japan’s Softbank and the United Arab Emrates MGX), which intends to invest $500 billion in AI infrastructure over the next four years. The intention, to secure America as the leader in the AI industry, and reinforce OpenAI’s position at the top. The day before, the tests for DeepSeek-R1, a Chinese-built large language model (LLM), were released, and in the days since its impact has shaken the US AI industry, with US tech stocks dipping as a result.
But what makes this new-comer so influential. The tests showed it merely being on a par with OpenAI’s smartest model o1, which is accessible through ChatGPT Pro for $200 per month. Despite this large cost Sam Altman (OpenAI’s CEO) claims that they make a loss on pro subscriptions. This is where DeepSeek steps in!
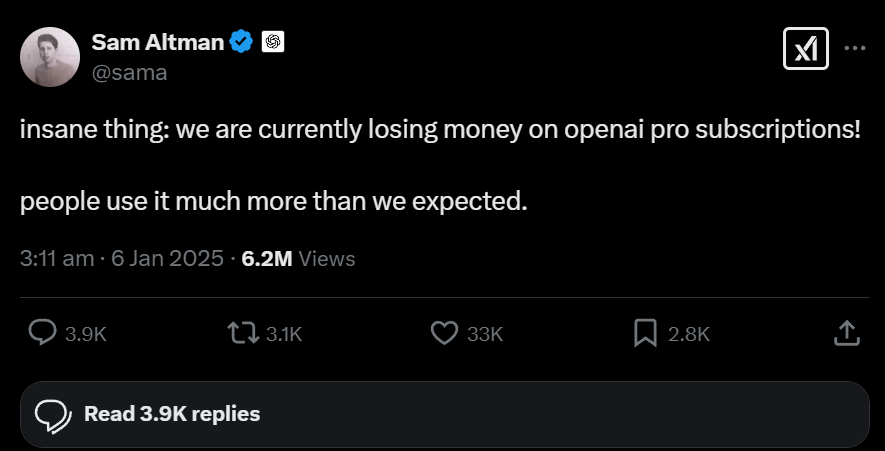
Unlike o1, DeepSeek-R1 is not locked behind a pay-wall, its free, and more importantly it is ‘open-weight’. This means that the algorithm behind the model is publicly accessible and can be downloaded for free and run locally, this is not the same as open source as the training data has not been made available. The openness of R1 has led to three million downloads of different versions of R1 being recorded by Hugging Face, the open-science repository for AI that hosts R1’s code. These downloads include versions already built upon by independent users, another benefit of being ‘open-weight’. This iterability could make it hugely influential to researchers, as building on the model will allow for it to be further refined to meet specific requirements, and allow many more individuals to play a role in improving AI models, thus taking away influence from OpenAI.
This is not the only aspect of DeepSeek that is causing a shake up; it’s cost to produce and run make it a game-changer in the AI space. Running R1 has been shown to cost approximately 13 times less than o1, according to tests run by Huan Sun, an AI researcher at Ohio State University in Columbus, and her team. Furthermore, it is believed that in training DeepSeek-V3 (the precursor to R1), High-Flyer (the company behind DeepSeek) spent approximately $6 million dollars on what had cost OpenAI over $100 million. R1 is based of the V3 model and is believed to also have been far more cost effective to train then OpenAI’s models.
So how is it so much cheaper? Well, as OpenAI’s o1 model is closed source how its model runs its not publically accessible, but it is believed to use ‘Mixture of Experts’, as well as ‘Chain of thought’ technique’s, these are also utilised by R1.
Mixture of Experts (MoE):
As LLMs are made up of vast networks, many questions will not utilise large sections of it, hence it is far more cost effective to only activate the relevant parts. This is what MoE does, with operations routing a question to the relevant part of the network, thus saving large amounts of computational power.
Chain of thought:
This technique essentially records the step by step process of solving a question and then uses these steps to come to a solution. This increases computational cost during the solving process but also improves the accuracy of results.
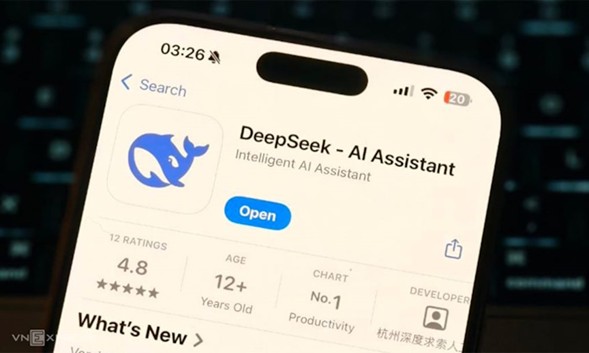
OpenAI likely trained o1 using Chain of Thought, through providing prompts, the desired thought process/internal monologue for o1, and then solutions; this is an example of where DeepSeek-R1 differs and saves money. R1 was trained using only prompts and answers, this requires vastly less data and allows the models internal monologue to emerge from the training process itself. Further costcutting likely resulted as R1 was built on Meta’s open-source Llama model, and there is evidence that it was trained using distillation of o1. This process uses outputs from larger, more capable models, to train and improve the preformance of smaller models, thus allowing simillar results to be achieved at far lower costs. If so the money pouring into the Stargate project to support the companies involved will likely allow smaller companies to piggy-back on their models and cost-cut in the training phase, thus calling into question the profitability of LLMs, and this likely caused the dip in American tech stocks seen last week.
Unless you work for any of the companies involved in the Stargate Project, DeepSeek is a major step in the right direction, drawing power and influence away from the growing monopoly of OpenAI, as well as enabling more rapid progress in the AI industry by providing access to its algorithms. This openness will also promote competition in this emerging industry, furthering its growth and technological advance.